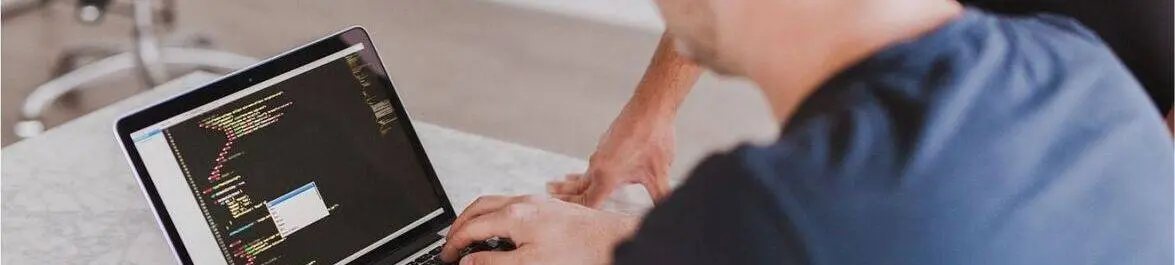
Preparing the Economists of Tomorrow
Encouraging collaboration in teaching data analysis skills
Read a summary using the INOMICS AI tool
Given the increase in data availability worldwide and the surge in demand for data literacy in the labour market, more and more economics courses are including data analysis skills in their intended learning outcomes. As a result, helping students to develop these skills in the classroom is increasingly important for economics educators.
We propose three tips on how to successfully encourage collaboration between students in learning data analysis for applied courses: (i) design engaging teaching materials, (ii) get students to work in pairs during classes, (iii) use peer feedback for formative assessments.
How to prepare: Engaging teaching materials
Figure 1: Cheat sheet used in a session on data analysis and visualisations in Python for complete beginners
First, when choosing the materials, it is key to use real world datasets that are relevant for your students and that they will be interested in exploring further. In this way, even the process of data cleaning, which is quite feared by many students, can turn into a more engaging exercise.
Second, during the applied classes (or labs), ask students to solve new exercises that they haven’t seen beforehand.
Third, it is important to design cheat sheets that students can use in class, to enhance autonomy during the sessions. This enables students to develop their technical skills and focus on the exercise, rather than spend the session struggling to discover basic commands they need to employ. A cheat sheet also acts as a revision aid for students later.
Thus, these cheat sheets should include the key commands (or functions) needed to tackle the exercises. Rather than making this a long list of commands, set up examples that students can follow easily.
Then, it is up to them to find the commands from the cheat sheet that are relevant to each exercise. We have included an example of a cheat sheet used during a session on data analysis and visualisations in Python for complete beginners (Figure 1). Students were provided the cheat sheet and given a set of exercises, using a completely different dataset.
How to deliver: Work in pairs in classes
To facilitate the use of cheat sheets, get students to work in pairs. Pair programming is widely used by data scientists in industry for coding tasks: two people use one computer, where the “driver” controls the keyboard, and the “navigator” observes and supports (Williams, 2001). The driver and navigator swap roles regularly, and discuss their work throughout (Demir and Seferoglu, 2021; Hannay et al, 2009).
Using this technique for data analysis encourages cooperation between students in the classroom, enhances learning by doing, and builds relationships between students. The teams can be set for the term, or they can change every class. What is important is that students work together and do not feel overwhelmed by the task at hand.
How to assess: Peer feedback formative assessments
Finally, use formative assessments (i.e., assessments that do not count towards the final course mark). These have been shown as central in promoting students’ learning and understanding (Wu and Jessop, 2018; Black and William, 2019; McMallum and Milner, 2021).
The challenge with these assessments is that many students do not engage with them and even if they do, many do not collect or incorporate the provided feedback in future assessments (Duncan, 2007). But evidence suggests that students find assessing the work of their peers quite motivating, enhancing their learning and development (Ballantyne et al, 2002; Vickerman, 2009).
Short regular formative assessments where students can offer peer feedback can be very easily incorporated into a course with a learning outcome of developing data analysis skills. First, students attempt the questions; next they submit their answers; finally, each student reviews another student’s attempt and provides written feedback. In this way, students get to see how other students have solved the questions, learning different ways to approach the exercises, while also checking the validity of the solution given by their peer and reflecting on the validity of their own proposed solution.
It is up to each teacher to provide guidelines for feedback, but we have found that it is best to initially give some guidance on what was well done and what could be improved, and to release the full solutions after the students have given feedback to each other. In this way students get to review the feedback received one more time after the answers have been made available, and reflect more when providing feedback to their peers.
Conclusion
While the task of working with and analysing data can be daunting for students at first, educators can make the learning engaging and more approachable for students by rethinking the design of the material, the delivery, and the assessments. Our tips get students to learn-by-doing and encourage them to be very active in classes, as well as outside the classroom when working on their assessments.
This article was produced in cooperation with the Economics Network, the largest and longest-established academic organisation devoted to improving the teaching and learning of economics in higher education. Learn more about the Economics Network here.
Header image credit: Pixabay.com.
References
Ballantyne, R., K. Hughes, and A. Mylonas, (2002). “Developing Procedures for Implementing Peer Assessment in Large Classes Using an Action Research Process.”, Assessment and Evaluation in Higher Education, 27(5), 427–41.
Black, P., and D. Wiliam, (2009). “Developing the Theory of Formative Assessment.”, Educational Assessment, Evaluation and Accountability 21 (1), 5–31.
Demir, Ö., and S.S. Seferoglu, (2021). “A Comparison of Solo and Pair Programming in Terms of Flow Experience, Coding Quality, and Coding Achievement.”, Journal of Educational Computing Research, 58(8), 1448-1466.
Duncan, N., (2007). “‘Feed-forward’: improving students' use of tutors' comments.”, Assessment and Evaluation in Higher Education, 32(3), 271-283.
Hannay, J. E., T. Dyba, E. Arisholm, and D.I. Sjøberg, (2009). “The Effectiveness of Pair Programming: A meta-analysis.”, Information and software technology, 51(7), 1110-1122.
McMallum, S., and M.M. Milner, (2021). “The Effectiveness of Formative Assessment: Student Views and Staff Reflections.”, Assessment and Evaluation in Higher Education, 46(1), 1–16.
Vickerman, P., (2009). “Student Perspectives on Formative Peer Assessment: An Attempt to Deepen Learning?”, Assessment and Evaluation in Higher Education, 34(2), 221-230.
Williams, L. (2001). “Integrating Pair Programming into a Software Development Process.”, Proceedings 14th Conference on Software Engineering Education and Training. 'In search of a software engineering profession' (Cat. No. PR01059), 27-36.
Wu, Q., and T. Jessop, (2018). “Formative Assessment: Missing in Action in Both Research-Intensive and Teaching Focused Universities?”, Assessment and Evaluation in Higher Education, 43 (7), 1019–1031.
-
- Research/Project Funding Opportunity
- Posted 1 week ago
Call for Research Projects 2025 "Women and Science"
at Paris Dauphine University in France -
- PhD Candidate Job
- Posted 1 day ago
PhD Student in health economics, epidemiology, health services research, public health, and/or migration
At Institute of Health Economics, Leibniz University Hannover in Hannover, Germany en de -
- Researcher / Analyst Job
- Posted 1 week ago
Applied Economist for Climate Risk Analysis
At Joint Research Centre of the European Commission in Sevilla, Spain