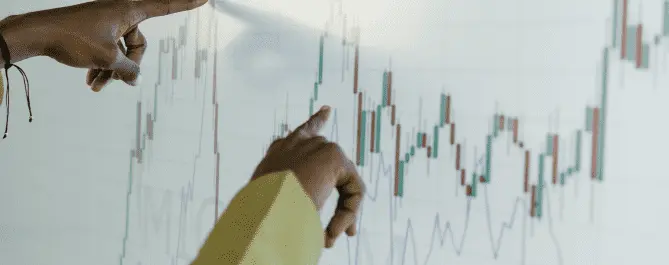
Study Advice
What is a "Model" in Economics?
Read a summary using the INOMICS AI tool
The word "model" is used a lot in discussions about economics. However, economics courses often don’t spend a lot of time defining the word or what exactly it means. Rather, students usually end up piecing together their idea of what models are after learning several specific examples (such as the Solow-Swan or OLG models).
This article fills in the gap by discussing the fundamentals of models and how they’re used in economics. There are many similarities between the myriad models utilized in the field, including common use cases and common assumptions. Understanding these commonalities will help you use and interpret economic models effectively, avoid misconceptions, and identify when these models are not necessarily relevant or useful.
The purpose of a model is to (more) easily study real life
Models in economics are imaginary constructs that approximate how the real-world economy functions, so that economists can predict how economic concepts and policies (would) work. Even though models are imaginary, they’re useful because each of them is composed of a set of rules and assumptions that attempt to reflect real-world behavior.
Many of these rules and assumptions are defined by math symbols. Once a model is fully constructed, economists most often use math to determine what the model ends up saying, or predicting, about the real world. These conclusions inspire most of the discussion and debate about economic models. Economists will often debate if the assumptions make sense, if a given model is accurate, and whether or not the model can help us learn about real life.
Because models are abstract, it’s very easy for economists to adjust them. This can be done by changing assumptions, or adding new ones. Often, new and useful models are built by adding onto older ones in this way.
Entire schools of thought in economics are often defined by this very activity. For example, when Keynesians decried the unrealistic assumptions of classical economic models (particularly the idea that all markets will self-correct and reach a socially optimal equilibrium, rendering government intervention virtually useless), classicists adjusted their existing models to account for more realistic behavior. This led to the “Neoclassical” school of thought.
It may seem like we’ve had plenty of time to build all of the economic models we need, but that’s far from the case. Theoretical economics papers are still routinely published, and economists are diving ever deeper into both theory and data to create models that can better approximate real life.
For example, macroeconomists continue to build macroeconomic models that directly flow out of microeconomic behavior, and microeconomists continue to build increasingly complicated models using learnings from fields like behavioral economics.
Models’ modular complexity makes them excellent for learning
Economic models are also highly useful for another important area: teaching. Economics students are often introduced to models like the aforementioned Solow-Swan and OLG models early on in their economics coursework. But, since models can include varying levels of complexity, students often revisit earlier models with added assumptions and more complicated constructions.
Revisiting models in this way allows a student to focus on the new complexities and implications, since they already understand how the model works generally. This makes models great tools for professors to use to demonstrate new theoretical concepts, and introduce more math and proofs to students.
Here’s a fun exercise: if you’ve studied an economic model in an economics class, search RePEc or Google Scholar for economics papers that discuss that model. Open one, and read the author(s)’ discussion of the model. You may be shocked to find that the model looks far, far more complicated than you remember from class!
Rather than confusing yourself, learning to interpret the more advanced versions of models (and compare them to what you already know) can give you a great glimpse into the frontiers of economic thought. And, you’ll find that many of the models’ assumptions remain either unchanged or are only lightly modified to allow for more realistic behavior.
In fact, many economic models include some key assumptions that you should become familiar with. Let’s dive into the most common ones and discuss their usual implications.
Common model assumptions to know
Perfect Competition
One common and important assumption that many models include is that markets are perfectly competitive. It’s important to master this introductory concept, because its implications are wide-ranging, and relaxing or changing this basic assumption often greatly changes model outcomes.
For example, perfect competition implies:
- Markets always clear.
- Goods are homogenous.
- Firms are “price takers”: they cannot set prices.
- Firms make zero profits.
- Firms utilize inputs to the point where their cost equals their marginal productivity; i.e., firms utilize labor to the point where marginal productivity of labor equals the wage rate.
Simplified goods
Very often when using a model, economists aren’t concerned with the exact goods that people buy. That’s because the specific products that people purchase usually don’t matter for the model’s outcomes. Instead, high-level concepts like overall income and utility – and how they relate to policies or macroeconomic phenomena like recessions — are more important.
To see this, imagine that you’re studying a model where individuals spend all their income to maximize their utility (a very common analysis!). In this construct, what matters is the total amount of utility the individual can purchase with their limited resources (typically represented by their income). We usually don’t care which exact goods make up that utility, though — whether it’s two dozen eggs, a steak, or a restaurant meal doesn’t really matter, because it doesn’t change our economic takeaways (unless, of course, we are specifically studying people’s food preferences — but in that case, we’d probably need real data, not a theoretical model!).
Because the specific mix of goods doesn’t normally matter, many models include some form of a simplified goods assumption. This means that we assume the economy produces only one or two goods, period. Further, this assumption usually states that these goods are homogenous (all the same), and fully sufficient for the economy and society to function normally.
This assumption is useful mainly because it simplifies the math, and lets economists focus on the meaningful high-level takeaways. This is easy to see by considering a Lagrangian Optimization problem that an economic agent might face. Economists solve these types of problems for many models, to determine how individuals will behave in that model, which in turn determines overall economy-wide outcomes.
Fundamentally, the budget constraint portion of these optimization problems must account for every price of every distinct item that the agent could purchase. In a model with one or two goods, the problem is easier and less unwieldy than if hundreds or thousands of goods were included. Moreover, abstracting away the myriad products available to us prevents nebulous concepts like preferences, brand loyalty, or cultural tastes from affecting the model’s outcomes.
Rational agents
The “rational economic man” is a straightforward fellow who lives in the world of economic theory. This rational agent is someone who always carefully considers all options they have, and chooses the optimal utility-maximizing decision every time. Rational agents therefore behave very predictably, and are quite helpful for economists attempting to describe how the world works. Assuming that all agents are rational (or at least that, on average, the typical agent is rational and thus economy-wide behavior will average out to rational behavior) allows economists to predict how — for example — certain fiscal policies might affect consumer behavior.
This is also helpful partly because assuming that people aren’t rational can be more complicated than it’s worth. It’s true that in real life people can make mistakes, and sometimes make irrational decisions. But, this is incredibly difficult to model in a theoretical economy. How people make mistakes, and when people behave irrationally, are most likely quasi-random events and would be much more complicated to model than people who always choose the best decision.
Further, from an economic theory standpoint, it’s usually not very helpful to allow for irrational behavior in models. When given a choice, almost every real person would prefer to make the best choice and avoid mistakes. If a bad decision ends up wasting money or time, that person’s utility suffers — but this doesn’t represent an interesting decision about allocating scarce resources. It was just a mistake that the person would, again, almost certainly choose to undo. Therefore, modeling mistakes or realistically irrational behavior likely wouldn’t change the model’s outcomes, and thus often isn’t necessary when constructing a model.
Representative agents
The term “representative agents” is often used when describing a model. In plain language, this assumption means that the model assumes everyone is the same. In combination with the rational agents assumption, most models therefore assume that every agent in the economy seeks to maximize their utility in a rational and identical way. Keep in mind that an “agent” in economics discussions can refer to individual consumers, producers, or households. Different models tend to focus on one or more of these entities and refer to them as agents.
The representative agents assumption is clearly unrealistic, but removing it wouldn’t change much about most models’ takeaways – just like the simplified goods assumption. In this case, assuming that everyone is the same means that economists can study how just one individual would rationally behave in the model, and then simply extrapolate or multiply that behavior onto an economy-wide scale.
If this seems ill-thought or lazy, consider that each representative agent can be thought of as the average of all other agents in the economy. This simply lets economists ignore the idiosyncrasies of every theoretical individual, and get to the pertinent takeaways.
Model assumptions (and omissions!) determine specific use cases
Many of the assumptions above seem very unrealistic or limiting. And, the justification for using them is often because they simplify the math and let economists get to a relevant takeaway without spending too long on minutiae.
While this may seem frustrating or obtuse, this fact hints at a very important concept: models have specific use cases where they are relevant and useful. Outside of these relevant use cases, a specific model probably should not be considered — or at least its findings should be used very carefully, if at all, and with a good portion of context and caveat.
In other words, specific models are made for a reason and used for specific purposes. If we know what those are, we can intelligently decide when and how to use the model, and when it’s best to set it aside. It’s easiest to illustrate this with an example.
Suppose that an economist wants to examine how, in theory, changing a pension plan might affect economic outcomes. Pensions are awarded to older employees once they reach the age of retirement.
First consider the Solow-Swan model, which features identical agents that live forever. In this model economy, each agent maximizes a utility function and chooses to purchase and consume a homogenous good; they also choose a portion of their income to save. This saving behavior could be useful when studying pensions, but agents that live forever don’t mesh very well with the idea of retirement: when would an immortal agent retire? That’s a strange — and not very useful — question to consider.
Next, consider the OLG model. It goes against the grain of many simpler models by including two different types of representative agents rather than one. It also states that agents live for precisely two time periods, when they are “young” and when they are “old”. These are very simple changes, but they create many layers of added complexity. This allows us to view how those two types of agents interact with each other, how they behave differently from each other and from themselves across time, and how having a life cycle affects their behavior.
Clearly, for the economist studying pension plans, the OLG model is the better choice. The specific assumptions it includes are far more helpful when discussing how pensions might affect economic behavior. In the OLG model, agents can work in the first period and retire in the second; but in the Solow-Swan model, they live forever and there’s no clear retirement age.
This illustrates another important facet of economic models: the assumptions and rules that they don’t include can tell us what to use them for, and when to set them aside. It’s clear from this example that the Solow-Swan model isn’t the best choice when examining pensions. It doesn’t include any assumptions about age or retirement, which should tell us that it was not intended to examine these concepts. The OLG model is much better suited to these examples, because it includes additional assumptions about aging over time.
That’s not to say the Solow-Swan model isn’t useful. It’s a very popular model for studying economic growth and savings more generally than the OLG model, because it doesn’t include additional complexity in the behavior of specific agents. This makes it a better choice for economists studying how fiscal policy might impact long-run economic growth, for instance, where the decision of when an individual should retire or how they might react to pension plans isn’t especially relevant.
Criticizing and debating models — the right way
In this way, each model tends to serve a set of general purposes, but they may not be very helpful when applied for study outside of those purposes. Economists understand this; many would quickly agree that a certain model does not realistically portray all of human activity. But, the vast majority of models don’t have to. They only need to realistically portray outcomes related to the specific use case they’re intended to help us study.
And, the modular nature of models means that economists can — and very often do — repurpose existing models to study their specific research question. Many economics papers include a section that identifies the basic model the authors intend to use, then introduces additional assumptions based on their research question.
Once a model has been defined, it’s very worthwhile to debate whether or not it makes sense for the task at hand, and if it models economic activity realistically enough to be worth using. Debates over model shortcomings are most productive when we consider what they’re used for.
But, beware of discussions about how unrealistic a model is when that discussion doesn’t acknowledge the intended use case. And keep in mind that even glaringly unrealistic assumptions – such as the Solow-Swan model’s infinitely-lived agents — don’t disqualify a model from use. They simply point to the proper use cases.
One last note: models in econometrics
The word “model” takes on a slightly different meaning in econometrics contexts. While the best econometric models are informed by economic theory, in regression analysis the word “model” often refers to the specific form of a regression model used.
There are many, many different types of regression models – gravity models, probit and logit models, Poisson models, ARIMA models, the famous OLS model…the list continues. These models all include and imply a set of assumptions and rules, just like more general theoretical ones do. But, in these cases, the assumptions and rules are more immediately math- and statistics-minded.
These models very frequently take a step beyond the theoretical world and utilize real-world data to take a specific form, then help the economist study whether or not an economic theory is supported by the real-world evidence.
Still, there are parallels between these two types of models. It’s important to learn about the assumptions of each type of regression model to learn when they can be used, and when their assumptions are too unrealistic for the task at hand – just like theoretical models. This requires a thorough understanding of econometrics, which can be a harrowing — but rewarding — journey!
In summary: models are abstract constructs that economists love to use. They’re helpful tools with proper use cases, but it’s important to consider what a model’s assumptions imply. And, each model has limitations that imply times when they should probably be disregarded.
Interested in learning more economic models? Check out our Economics Terms A-Z, or take the plunge and look for a course of study yourself!
-
- Professional Training Course, Supplementary Course, Online Course
- (Online)
- Posted 10 months ago
Introduction to Data Science in Python
at Coursera -
- Programma di Dottorato
- Posted 2 weeks ago
Doctoral programme in Economics (MRes+PhD)
Starts 1 Oct at Economics, University of Surrey in Guildford, Regno Unito -
- Programma di MBA
- (Online)
- Posted 4 years ago
Master of Business Administration (International)
Starts 30 Jun at University of Tasmania in Hobart, Australia